Machine Learning in the Life Sciences Has a Data Problem
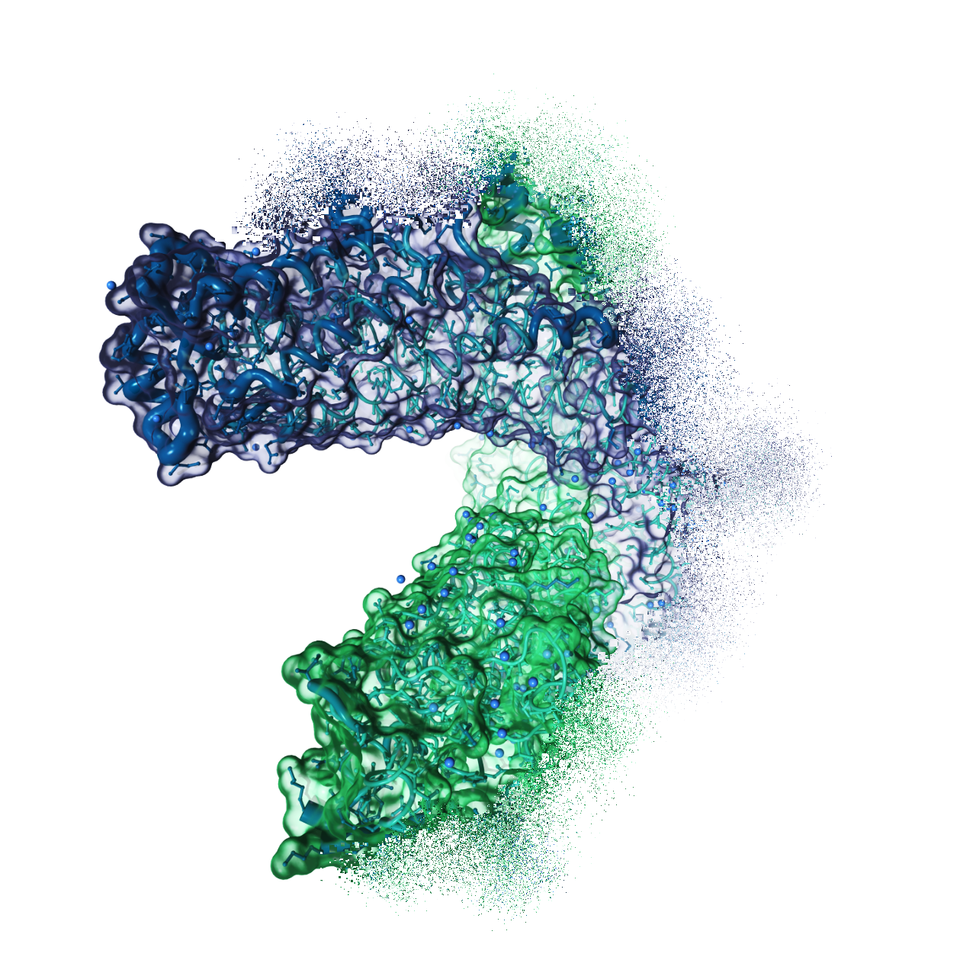
The world is captivated by the unrelenting progress of generative artificial intelligence (AI). Yet amidst this technological revolution, not all sectors are poised to ride the wave of AI-driven advancement.
One such area is the life sciences, where despite a vast landscape ripe for the application of machine learning (ML), the promise of transformation remains largely unfulfilled. The crux of the issue lies not in the lack of opportunities, but in the quality and accessibility of data. To truly unlock the potential of ML in the life sciences, better approaches to data acquisition, management, and distribution are needed.
Artificial Intelligence in the Life Sciences
The intersection of AI and life sciences has been an area of intense interest for decades. Fields such as genomics, medical imaging, disease diagnosis, and drug discovery have all experimented with machine learning in a bid to uncover patterns and insights beyond the reach of traditional methods. Yet for all the promise and hype, the reality of AI's impact on the life sciences is a story of varying success. While there have been noteworthy achievements, the overall landscape is more complex.
The crowning achievement of AI in life sciences to date is indisputably DeepMind's AlphaFold, a deep learning algorithm that predicts 3D protein structures with unprecedented accuracy. So how did AlphaFold succeed in a domain where other applications of AI have faltered?
While the triumph of AlphaFold rightly belongs to DeepMind, the key to its success is rooted in a globally coordinated effort to compile extensive, high-quality, and publicly accessible protein databases.
DeepMind trained AlphaFold on a vast collection of protein data, curated by institutions like the Worldwide PDB, the US's RCSB and Europe's EMBL-EBI. These entities have made it their mission to freely disseminate this data to the global community, laying the foundation on which innovations like AlphaFold could thrive. Protein data banks exemplify an unusual level of data sharing in the fields of chemistry and biology. AlphaFold stands on the shoulders of countless PhD students and scientific researchers, who toiled to crystallise proteins, decipher their structures, and share the results with the global scientific community.
The story of AlphaFold underscores a pivotal challenge in the wider application of AI in life sciences: the need for comprehensive, high-quality datasets. Such datasets are a rarity in most domains.
The Problem with Public Life Sciences Data
One of the most alluring sources of publicly accessible life sciences data is the ever-growing corpus of published scientific literature. As long as scholarly papers have been written, there have been efforts to mine useful information from them. AI is now establishing itself as the frontier tool for extracting and leveraging scientific insights.
The lifecycle of published research, and how it gets consumed by machine learning algorithms, can roughly be summarised as follows:
- Scientists around the world conduct a multitude of largely uncoordinated experiments, often employing non-standardised systems and protocols. These experiments output simplistic results, designed for straightforward human interpretation rather than machine-readability.
- A slither of these experiments yield positive results, deemed interesting and worthy of publication. Many of these results are irreproducible, and a handful may even be intentionally falsified. The bulk of experiments—those yielding negative results—never see the light of day.
- Of these select experiments deemed publishable, only a small portion of the corresponding data gets reported—often, just the final result.
- The experiment gets transcribed into natural language as confusing academic prose, and other machine-unfriendly, inefficient forms of information capture.
- Eventually, possibly years later, the paper reaches the broader community, albeit likely behind a paywall. The underlying data is rarely shared, but some papers may make the false promise that "data will be made available upon reasonable request".
- AI companies ingest these papers and attempt to extract useful data using natural language processing (NLP) and computer vision (CV), inevitably with some inaccuracies and information loss.
- This incomplete, biased, low-quality data becomes the foundation for applications of machine learning in the life sciences.
Clearly, this scenario is far from ideal. Scientific literature, while seemingly a treasure trove of knowledge, presents a dataset that is inherently flawed for machine learning.
The Problem with Private Life Sciences Data
Pharmaceutical companies, with their proprietary datasets, may seem to sidestep many of the issues associated with public research literature. However, they present their own unique challenges:
- Much of industrial scientific experimentation is still done by hand. Whilst lab automation is on the rise, the complexity of implementing robotics for dextrous tasks limits automation to narrow domains like high-throughput screening. This has implications on both the volume of experiments that are conducted, and the breadth of data collected from each of them.
- Many pharmaceutical companies continue to rely on electronic lab notebooks (ELNs) and laboratory information management systems (LIMS) that are outdated and cumbersome to use. Some of these legacy systems are so disorganised and technologically backwards that they're borderline impenetrable to engineers hoping to access usable data. These antiquated systems often deter scientists from capturing data in a structured format, instead preferring to write shorthand notes that are difficult to extract meaning from or lost entirely. Many of pharma's most precious insights are trapped inside PowerPoint slides.
- As is the case for published research, experiments are designed with human interpretation in mind, not machine learning applications. Instead of retaining the complete data collected by analytical machines, it's common for only highly condensed results to be captured.
As such, while private datasets may provide a solution to some of the challenges faced by public research, they too are far from perfect.
Biology's "Next Word Prediction"
For Natural Language Processing (NLP), the simple mechanism of 'next word prediction' has proven astonishingly effective. It turns out that training a model to predict the next word in a sentence scales impressively, and generalises surprisingly well to most text-based tasks. The breakthrough of ChatGPT is not owed to a fancy algorithm: it's the result of immense computational power directed at massive datasets in the right way.
A similar story applies to generative AI for images. Diffusion models start from random noise and gradually transform it until a coherent image emerges. This simple idea produces outstanding results, providing you feed the algorithm with enough data.
In contrast, the life sciences haven't yet found their own 'next word prediction'. Such a mechanism would ideally be a simple and universally applicable ML approach that, when supplied with enough high-quality life science data and trained with ample computational resources, would yield remarkable results for tasks in chemistry and biology.
A mechanism like this is by no means essential. AlphaFold didn't rely on such a universal 'trick'. Instead, it used a bespoke neural architecture with distinct modules, each crafted for specific aspects of the protein folding problem. Nonetheless, discovering a universal mechanism could propel the life sciences into a new era, potentially revolutionising the field as profoundly as 'next word prediction' did for NLP.
Addressing the Challenges
While it's simple to state solutions to these problems, implementing them is decidedly more complex.
To start, scientific publishing practices could clearly be improved. We should encourage the publication of negative results and reward people for reproducing important findings. Moreover, a culture of sharing data and code should be fostered. That said, shifting the entrenched incentive structures of the academic publishing industry and encouraging open sharing—especially when it doesn't serve the author's own interests—is no trivial task.
As robotics matures, there will be a tipping point where comprehensive lab automation becomes both technically feasible and economically viable. This will be coupled with more exhaustive data collection and better data management systems.
Companies such as Recursion and Insitro are already leading the charge towards a future where drug discovery is driven by data designed for machines; not humans. In the extreme, one might hope for an 'AWS of biology' to emerge, democratising access and commoditising lab experimentation. This softwarification of biology will naturally lend itself to ML-centric approaches.
The advent of cloud biology could also catalyse open science in ways that were previously impossible.
If there is a 'next word prediction' equivalent awaiting discovery for biology, open sourcing the scientific process could be the key to uncovering it.
The collective intelligence of the global scientific community could provide the fertile ground needed for such a breakthrough.
Finally, the proven success of protein data banks offers a glimpse into the future, underpinning the potential of initiatives aimed at disseminating high-quality life sciences data. This alone won't spark a revolution, but it will provide the much-needed fuel for ML's next breakthrough. The true transformation of life sciences through AI hinges on the convergence of multiple innovations—lab automation, sophisticated data systems, open source science, and more. Get the datasets rights, and it becomes the foundational bedrock upon which these innovations will flourish.
Member discussion